Names and What They Say

An investigation into names, trends, and what they reflect
GitHub Gist | Interactive Jupyter | PDF Notebook
It has been a while since I becameas enmeshed with a set of data as I did with this one. While a huge part of me repeatedly found places where I could easily call the project complete, places where if I had stopped, there would be so much interesting information to investigate in this article, another part of me kept imagining other inferences that could be made. And I could not resist the urge to continue manipulating the data. I reached a point, however, where I realized that if I did not force myself to stop I would have to commit to a much larger-scale publication of this project. So I figured it was time to start writing about what I have found. Here we go!
So what is this data that I found so enthralling, you ask?
The Data
The data that makes up this project comes from the US Government's www.data.gov website, which contains a huge amount of data from many different government agencies. This project is specifically focused on their Baby Names data. The downloadable zip file at the bottom of this screenshot, as of today, contains 142 files, a different txt
file for each year starting in 1880 up through the most current, which is now 2021.
One limitation to keep in mind is that a name is not accounted for in this data unless its total count in a year reaches 5. The data collected near the beginning of the time range is also possibly skewed, considering that not all people born in the US were registered with the government.
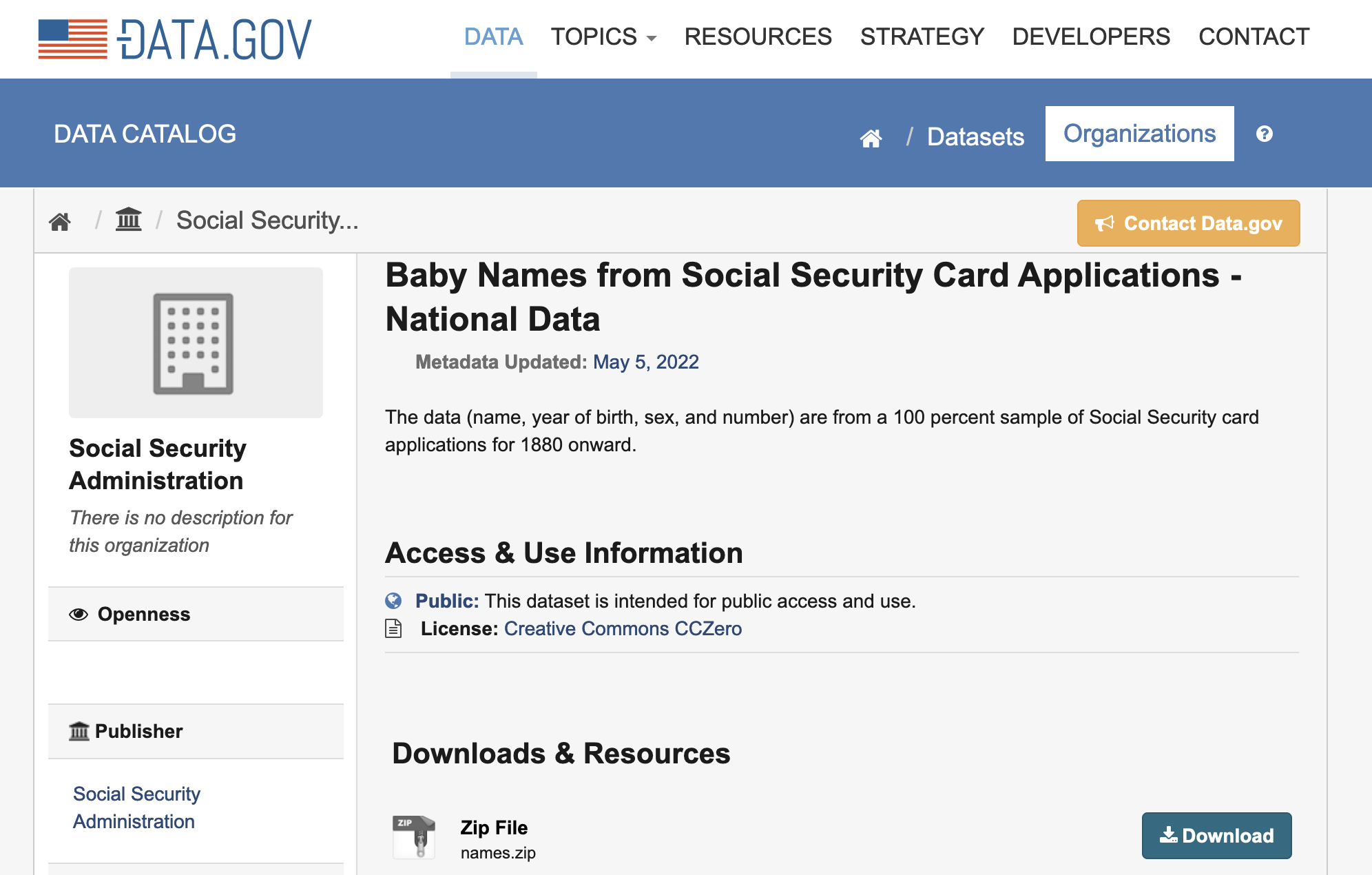
Each text file contains all of the names of babies who were registered at birth that year, the gender they were born with, and the count of how many of that particular name and gender combination were born that year.
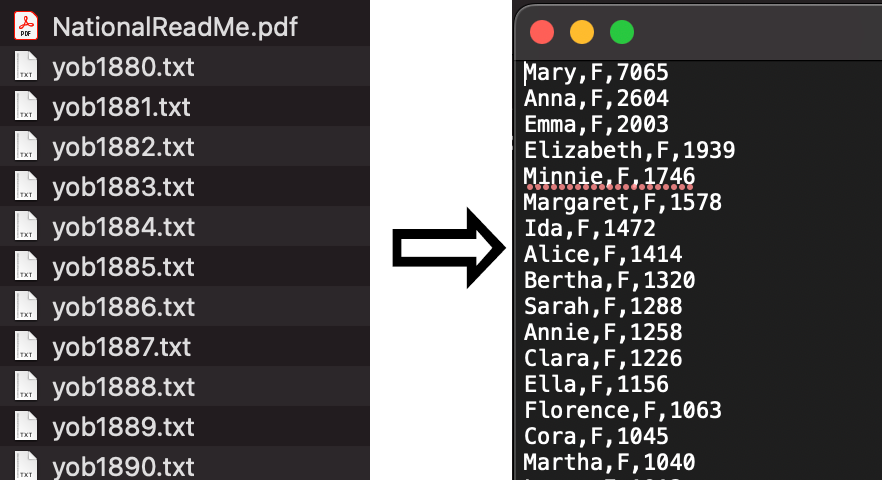
This seems like incredibly simple data. And in essence, it is. But it is amazing what you will find within it. Aside from the seemingly infinite social inferences I was able to find in this data, more impressive was the sheer number of features that could be engineered from just 3 columns of data. So let's get investigating!
Acquiring and combining the data
Firstly, as you might have noticed, the data comes in 142 different files, one for each year represented in the overall data collected by the US government. That means there is a little administrative work which must be done first. These cells show all of the necessary libraries for this project, the retrieval and extraction of the zip file, and the process of looping through all the txt
files and creating dataframes from each.
The next step is concatenating every one of these 142 files into one combined dataframe that still reflects the year, which originally was only labeled as a part of the txt
file name. The following cells show the process of combining all of the dataframes into one using pd.concat()
The next step was to work on reducing the memory footprint of the data as much as possible. The one column that can be optimized is the gender column, since it will only ever contain an "M" for male or an "F" for female. So I converted this column to categorical data, which reduced the memory necessary from 62.9 MB to 48.9 MB. It might not seem like much, but when you are waiting for a function to finish aggregating that much data, that is a lot of minutes you can save!
Initial data investigation
And this is where the fun begins! We can finally start looking at the data in a more real way and start finding all sorts of trends and inferences. Firstly, we can get a look at the overall totals in the data:
The dataset has a total of 2,052,781 name and year combinations.
There are 142 different years represented in the dataset.
There are 101,338 different names represented in the dataset.
The following cells contain the code that arrives at the above totals, as well as the top 5 names by overall count for females and for males as represented in the data.
These cells represent the names that appear most frequently in the dataset.
This is data visualization of the total number of babies born and of the number of unique baby names each year over the course of the entire time range. This gives us a good idea of population growth as well as the growth in uniqueness and individuality within the names. It reflects a growing diversity in the US population, as you will soon see laid out.
Adding popularity and ranking columns
Adding a popularity score and a ranking for each name will give further opportunity for comparing names and their popularities across time, fluctuations in the popularity of individual names, and will give us further insights.
Here, I am using popularity and ranking columns as well as a custom function to see the most popular names for any given year.
name_filter()
takes a name and gender and returns the data on that combination.
plot_popularity()
takes a name and gender as user inputs and plots the popularity over the years for that name. This function and others like it will be instrumental in further data investigation.
how_many_ever()
is a function I wrote to calculate the total number of people of a given gender who have been given a specific name, as provided by user input. The result is accompanied by an image reflecting the relative popularity of the name and gender combination. The following cells show the results for the name "Chester" and how it is represented by females over the time range. The concept is growing on me!
popularity_vs_ranking()
is a function that will plot not only the popularity, but the corresponding ranking for a given name-gender combination. It is interesting to see how the popularity over the years and the resulting ranking inversely correspond. This is especially interesting for names like, in this example Linda, which spiked in popularity at one point and then quickly died away again.
plot_subplots()
is a function I will be using for the rest of this project to plot the trends for groups of names. Here is the code for this incredibly useful function.
Popular names across the time range
Now it is time to look at groups of names and how they have fared across time. First, we will look at the top names for females and males. In order to get those names, we must make the following calculations. And the accompanying function, listify()
will turn those results into a list of tuples, each containing a name a gender, which can be fed into plot_subplots()
to visualize the results.
The following are the top 4 names for males and females and their popularity visualizations. Many of the most popular names are ones that were extremely popular at the beginning of the time range and have made a comeback in recent decades or years. There are the rare few which have stayed popular across the entire time range.
Changes in name popularity
In order to further evaluate the changes in the popularity of names over the years, I added three more columns: popularity_last_year
, popularity_difference
, and percentage_difference
. These will allow further investigation into trends and changes in a more fine-tuned manner, giving access to the changes from one year to the next. This will help pinpoint more specific moments of popularity spikes and variations in the uses of different names.
Here are the top names with the sudden largest increases in popularity. Some of these names seem to come out of nowhere, never having been found in the records before and appearing one year. Others experienced lower popularity and then had sudden popularity spikes.
Likewise, there are names that experience a sudden and rapid decrease in popularity. Here are the top names from that category.
Famous names and other name trends
At this point in my data investigation, I began noticing some very interesting trends. Aside from Biblical and other religious names which tend to stay popular across the time range, it became clear that there were very specific spikes in name popularity due to popular cultural influences and shifts in demographics. So this section will dive into some of these very interesting social trends.
The groups of names I chose to include in this investigation are: celebrities names and how they encouraged popularity, notorious indiviuals and how they discouraged the use of names, names that are also the titles of songs and how they influenced immediate popualrity of a name, slavic names reflecting the influx if immigration centered around the fall of the Soviet Union, Cuban and hispanic names and how they spiked around the time of mass immigration, and Jewish names, reflecting the immigration of Jewish individuals into the United States.
First, let's look at names of famous people and how they affected the name trends and popularity. I was quite surprised by how much popular culture can play a part in something so important as the naming of a child for so many. Some of these spikes are a little hard to pinpoint just why they happened when they happened. So let's look at the 22 celebrity names included in this investigation, when they spiked in the data, and what the correlating factors might be.
Famous names (visualized below)
- KELLEN - popularity spikes ar0und the early 1980s and again around the early 2010s. The popularity can be traced to the NFL careers of Kellen Winslow, whose career spanned from 1979 to 1987, and his son, Kellen Winslow II, who was originally drafted to the NFL in 2004.
- MONTANA - popularity spikes in the 1990s and again in the most recent years. The popularity of this name can be connected to the successful career of football star Joe Montana. The later popularity of this name is most likely due to the popular television series Hannah Montana, which ran from 2006 to 2011.
- BRITNEY - popularity spikes in years 1988 and then again around 2000. The second spike in popularity of this name closely coincides with the popularity of recording artist and celebrity Britney Spears, whose career took off around 1997.
- WHITNEY - popularity spikes in year 1987 and continues for almost 10 years. This closely coincides with the success and popularity of recording artist Whitney Houston.
- SHIRLEY - popularity spikes in the 1930s. The popularity of this name closely coincides with the popularity of the child star, Shirley Temple, who was born in 1928 and was Hollywood's top star from 1934 to 1938.
- PRINCE - small popularity spikes in 1980s and large spike starting around the time of the death of the recording artist known as Prince.
- BEYONCÉ - never appeard in the data until 1998, and then popularity spikes in year 2000 and stays active for about 10 years. The popularity of this name closely coincides with the success and popularity of the recording artist.
- TAYLOR - popularity spikes in year 1995, but surprisingly does NOT spike in correlation to Taylor Swift's career, which given other trends, could be viewed as surprising. I keep this name in the visualizations as a sort of interesting outlier, given the fact that Taylor Swift has had such a large and sustained impact on popular culture.
- OPRAH - popularity spikes just after 1986. The popularity of this name closely coincides with the success and career of superstar, Oprah Winfrey.
- BARACK - popularity spikes around 2008. The popularity of this name closely coincides with the election and presidency of Barack Obama, the 44th president of the United States and the first African American president.
- KEANU - popularity spikes in year 1991. The popularity of this name closely coincides with the career of American film star, Keanu Reeves, whose first popualr film was Bill and Ted's Excellent Adventure in 1989.
- ANGELINA - popularity spikes in year 2000. The popularity of this name closely coincides with the growing popularity and success of actress Angelina Jolie.
- ADELE - was very popular at the beginning of the time range followed by a long decline, but then popularity spikes again in year 2010. The popularity of this name closely coincides with the popularity of the recording artist.
- KANYE - name comes out of nowhere, appearing first in 2002, and then popularity spikes in year 2004. The popularity of this name exactly coincides with the year of Kanye West's debut album.
- MARIAH - popularity spikes in year 1990. The popularity of this name closely coincides with the popularity and success of recording artist Mariah Carey.
- SELENA - popularity spikes in year 1995 and trends upward again around 2015. The popularity of this name coincides with two celebrities, the death of recording artist Selena in 1995 and the popularity and success of recording artist and actress Selena Gomez.
- KOBE - comes on the scene in the 1990s and popularity spikes in the early 2000s and then in the past couple of years. The popularity of this name exactly coincides with the success of L.A. Lakers basketball star Kobe Bryant, which ran from 1996 until 2015. Kobe Bryant was killed in an accident in 2020, which could account for the spikes in popularity recently.
- SHAQUILLE - popularity spikes in the early 1990s, mostly from 1991 to 1996. The popularity of this name closely coincides with the early success of basketball superstar, Shaquille O'Neal.
- CHER - popularity spikes throughout the 1970s. The popularity of this name closely coincides with the early career and success of Cher, particularly of her television show, Sonny and Cher, which was incredibly popular during the 1970s.
- DENZEL - popularity spikes in the early 1990s. The popularity of this name closely coincides to the rise in popularity and success of film star Denzel Washington, who began receiving academy award nominations for his films at the very end of the 1980s and then made a huge name for himself in Spike Lee's Mo' Better Blues, followed by Malcolm X around the exact time of the spike in name popularity.
- REESE - name comes on the scene for females just before 2000, and then rises in popularity and stays steady up through current time. The popularity of this name precisely coincides with actress Reese Witherspoons film breakthrough came in 1999 with the film Cruel Intentions.
- ELVIS - popularity spikes in year 1955. The popularity of this name directly coincides with rock n' roll idol Elvis's first recording contract.
Notorious Individuals' Names
- HILLARY - had been gaining popularity thoughout the 1980s and very early 1990s, but then falls off a cliff right around 1993. Hillary Clinton was first lady, wife of 42nd US President Bill Clinton, whose years in office spanned from 1993, the year the name Hillary plummeted and continued to decline from there, to 2001.
- ADOLF - had been dwindling in popularity over the 1920s and 1930s then almost disappears around 1940. The sudden decline can easily be tied to German dictator Adolf Hitler, with whom the Allied Forces, including the United States, fought World War II from 1939-1945.
- OSAMA - had been gaining popularity from 1968 up to 2001 and then drops immediately following 2001. This was an interesting one, because the name had been rising in popularity, probably due to increased immigration to the US from the middle east. But immediately following the 9/11 attacks on the World Trade Center in New York City in 2001, for which Osama Bin Laden claimed credit, the name almost disappears.
- SADDAM - first appears in 1990 and then decreases and disappears after 2 more years. This one was also strange, because the Gulf War in which the US fought Saddam Hussein, was fought from 1990 to 1991. The name Saddam never even registered on the radar with the US government data until 1990, with a low but existent popularity score. It is then followed by descreasing yet suprisingly present appearances two years following and then never appears after that. It begs the question, why?
Song Name Names
- JOLENE - popularity spikes in 1974, the same year country music legend Dolly Parton released her award winning song of the same name.
- RHIANNON - appears in 1974, and popularity spikes by 1976, the same year the award winning song of the same name was released by Stevie Nicks and Fleetwood Mac.
- SHARONA - popularity spikes in 1980, one year after the incredibly popular and iconic song, My Sharona, was released.
- ADIA - popularity starts to go up around 1995 and hits a high around 1999. The popular song of the same name was released in 1997 by recording artist Sarah McLachlan on her fourth studio album.
- FANCY - popularity spikes in the early 1990s. This one is a little different. It was never an incredibly popular name, but there is a verifiable spike in popularity the same year country music legend Reba McEntire released her recording of the song of the same name and quickly hit the top ten. The song was originally written and recorded by Bobbie Gentry in 1969.
- BRANDY - popularity spikes around 1973 and continues for about 10 years. This names spike in popularity follows the year the song Brandy (You're a Fine Girl) by The Looking Glass was released. The song was the qunitessential one-hit wonder, as it topped the Billboard Hot 100. But it was the only hit the band ever had and one of only three singles they ever released.
Pop Culture Character Names
- HAN - appears in 1967 and starts to spike around the late 1970s. Popularity gains new momentum around 2010. This popularity coincides with the first appearance of the character Han Solo in the Star Wars franchise in 1977. The character reappears in 2015 in The Force Awakens, after 32 years following his last appearance in a Star Wars film.
- LUKE - another Star Wars phenomenon, Luke had almost completely died out but made a huge comeback at the beginning of the 1980s and has stayed popular ever since. In spite of other Biblical names remaining fairly popular across the time range, this one stayed pretty low key until the 1980s. This popularity coincides with the first film of the Star Wars trilogy in 1977.
- EMMA - was extremely popular at the beginning of the time range, steeply decreasing and staying low in popularity until the mid 1990s and 2000s, when it regained momentum. Many believe this particular renaissance of the beautiful name Emma is due to the 2002-2003 baby character of the same name on the hit show Friends. I personally just think it is a beautiful name and named my own daughter Emma...no connection to Friends whatsoever.
- BAMBI - spikes over the mid to late 1950s and through the early 1960s. The Disney film Bambi was released and played in theaters in the years 1947, 1957, 1966, 1975, 1982, and 1988. It looks like the 1957 and 1975 releases could have had an impact on baby names. It is surprising, however, the dip in popularity during the same time as the 1966 release.
- BUFFY and JODY - both of these names have significant spikes in the year 1967, which was the year following the premier of the popular television series, Family Affair, in which two of the children characters are named Buffy and Jody. The show ran until 1971, which coincides with continued popularity before a falling off.
- BETTY - starts to trend upward in the mid 1910s and hits its peak in 1930, directly coinciding with the popular icon, Betty Boop, a cartoon with 90 theatrical productions between the years 1930 and 1939. Following the curve for the name Betty, one could speculate that Betty Boop possibly caused the decline in the name before completely falling out of popularity, as it had been on an incline and peaked the same year Boop came on the scene.
- KATNISS - appears in the year 2012 and peaks in 2014. The popularity of this very strange and new name directly coincides with the film series, The Hunger Games and its very popular main character, Katniss Everdeen
- BARBIE - trends upwards slightly in the late 1950s and then peaks around 1960, directly following the first release of the classic American doll, Barbie.
- KEN - hits a large peak the early 1960s, directly coinciding with the release of the Ken doll. The name Ken does not enjoy the same trend as the name Barbie and dies off much sooner than his counterpart.
History in data trends: Slavic names
The official fall of the Soviet Union occurred in 1991. There was a great deal of immigration before the actual fall of the union, but following the fall, there was an extremely high immigration of Russian and slavic people's into the US, as is clearly represented in the following data for Russian and slavic origin names.
History in data trends: Jewish names
Before the fall of the Soviet Union in 1991, in the year 1971, the Soviet Union lifted its ban on Jewish emigration, allowing those of Jewish faith and heritage to leave the Soviet Union. While many left for Israel a large number came to the United States as is reflected in the data below for primarily Jewish-origin names.
History in data trends: Cuban and hispanic names
The Cuban Revolution took place over the years from 1953 to 1959, during that time the difficulities of life in Cuba pushed many of its citizens to flee. The Cuban population in the United states rose from 79,000 to 439,000 by 1970. Many of the following names are popular Cuban names and appeared with very low if any frequency in the US before the immigration of Cuban refugees. A number of these names are also popular across other hispanic cultures as well, but the Revolution and its consequences seem to make a very clear appearance in the popularity of these names within the data in spite of other previous hispanic immigrations.
Unisex names
Calculating the popularity of unisex names in a meaningful way takes some extra steps. First of all, I categorize unisex names as being a name that does not have a NaN
value in either gender column, hence is represented by both males and females. In order to do that, I must first convert any 0
values to NaN
values.
The next step is sorting the names in the order of least difference between the counts of male gender use of the name and female gender use of the name.
Next, I chose the cutoff as 15,000 for the lower count gender use of the name in order for a name to count as unisex. For example, I am a female named Evan. But my name is not as much a unisex name as a name that can be creatively used to name female babies, thereby enforcing a sense of independence and perserverance throughout her life...or maybe I am just talking about myself. Needless to say, there has to be a cutoff somewhere, and it seems 15,000 for the overall count would be a good minimum to truly consider a name as being unisex.
Next, I order the names that have met the above criteria by how much of a difference there is between the higher represented gender and the lower. The closer the two are, the more unisex a name is. These are the highest ranking names and how far apart their representation in the data is, followed by a dataframe representing the counts of these top unisex names for each gender, and finally a side-by-side visualization.
Aggregated data investigation
To get a new perspective on the data, more than just how popular a name is or how high or low its ranking was in any given year, we can use an aggregated form of the data and investigate further. The following cells explain the columns in the aggregated data and contain the code and sample of the aggregated data.
The following is an aggregation dataframe for the names that have the most popularity overall in the data. This allows us to get a deeper understanding of the data for these names and investigate further.
With the aggregated data, it is possible to add another column for further analysis, a variation score column, which will give us insight into names that remain steady at whatever level of popularity they may weigh in, versus names that experience more fluctuation in their level of popularity.
This is an interesting investigation, because even the names that experience the lowest level of variation can experience some huge changes in their levels of popularity. But they tend to remain at a level of popularity, or at least in the same vicinity, over long periods of time.
Closing
This was by far one of the most addictive, fascinating, and eye-opening data collections I have worked with, simply because there is so much that can be directly seen or otherwise inferred from this data. Their are statements about what the United States means as a whole, the people who make up this country, the trends we tend to follow, the demographics, what we are made up of, what is important to us, and honestly, who we are. The naming of a child is one of the most important decisions a human can make. It is something that usually a person keeps with them their whole life, and even if they choose to change it, the name they are given at birth is never forgotten. So the results of so many millions of decisions made tell us not only the facts of the names of Americans but also what drives the individuals who chose those names. It is fascinating. And like I said in the beginning, I can either stop here and be happy with a fantastic project, or I could spend many more hours with this data and find out even more. For now, I will be satisfied with the project where it is. But I could definitely see there being a sequel to this little trip. It was quite an enjoyable one. Thanks for joining me! Happy data wrangling!